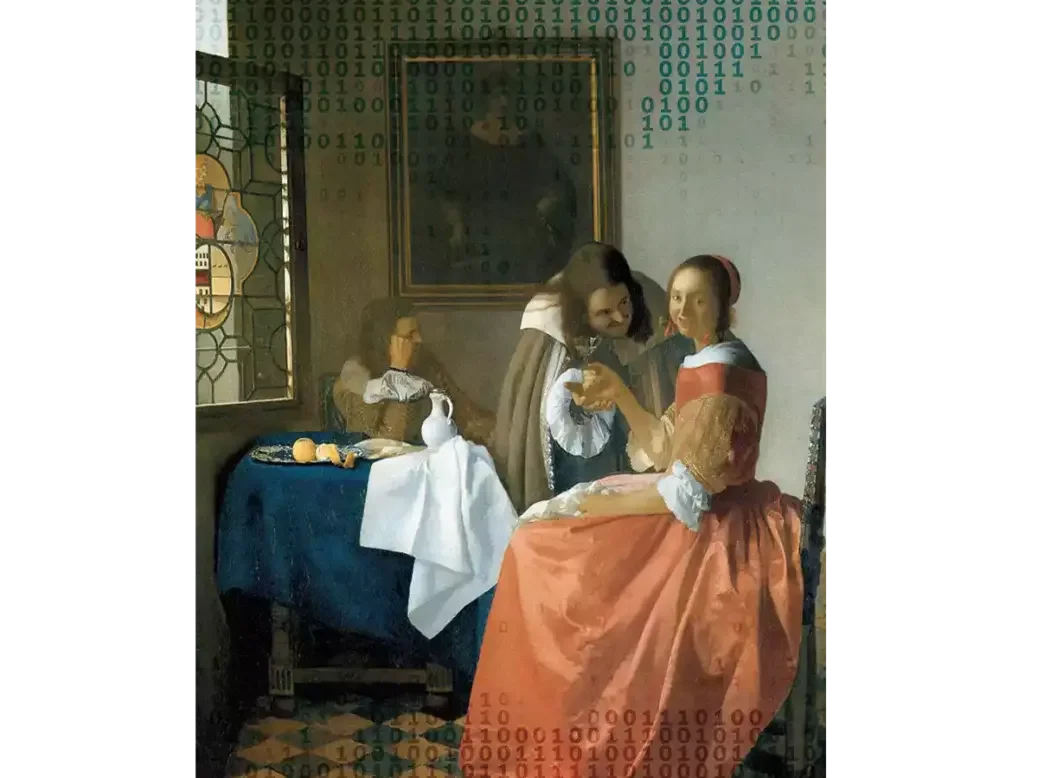
Professor Barry C Smith explores the philosophical implications and practical possibilities, for good and ill, of the increasing use of AI in the wine world.
Scarcely a week goes by without us hearing of an advance in artificial intelligence or a new application for it, whether it be medical breakthroughs in interpreting X-rays, DeepMind’s protein folding AlphaFold leading to drug discoveries, or the astonishing, humanlike performance of large language models (LLMs) like Open AI’s Chat GPT or Google’s Gemini. It is all the more remarkable to realize that LLMs can generate screeds of relevant and articulate prose when all they have been trained to do is predict the next word or token in a sequence—albeit in a highly constrained algorithmic way. Perhaps auto-complete is cleverer than we thought, or maybe our ability to string words together into sentences is just another form of auto-complete.
However we measure up in this new digital age, an area of human accomplishment that appears to be beyond the reach of the current AI revolution is wine tasting. But will this always be the case? Would we bet on it? The odds are shortening, and we cannot rule out advances in automated systems having a significant impact on the world of wine. At the same time, we need to be wary of the hype surrounding this second wave of AI. Therefore, it’s a good time to take stock of where we are and what might be coming.
To review the current state of play, we need to be as clear as we can about definitions and avoid applying the term AI to every kind of digital technology. Not every app or algorithm is powered by artificial intelligence. Some merely aggregate data and are incapable of generating new insights or of improving decision-making. By contrast, AI systems go well
beyond the big datasets on which they are trained, finding patterns in that data by means of machine-learning algorithms that learn from examples. These patterns enable the system to extend its application to novel cases, allowing it to model things like the underlying structure of folk songs, the likelihood of prisoners re-offending, or a consumer’s creditworthiness. In these and other cases, artificial intelligence is about digitally creating, in software tools or robots guided by software, capacities we thought only humans were capable of exercising. AI is not always limited, however, to modeling aspects of the human world. It can also be used to create large statistical models for predicting the course of a disease or improving long-range weather forecasting. The latter can be useful to winemakers and is already playing a peripheral, though important, role in viticulture, as part of AI’s wider contribution to agriculture: from satellite field and soil inspection, to plant health and fruit-picking decisions, to sorting and grading.
The more fundamental concern, however, is whether AI will ever be able to replicate human involvement in wine—a person’s expertise in tasting and appreciating a wine, their identification of the vintage or maker. Could a well-trained AI system end up exceeding the competence of wine professionals who make, select, and write about wines?
VR wine experiences?
There’s reason to be doubtful when it comes to wine tasting. The act of tasting involves smell, taste, and touch—three senses missing from the lives we live online. Tech entrepreneurs are wont to promote the fantasy that since we spend so much of our time in front of screens, we will one day be able to create an entirely digital record of our lives in files uploaded to the cloud. Will we, though? We would do well to remember that the recorded chapters, like the worlds we vicariously inhabit at the cinema, will consist entirely of sound and vision. These are worlds devoid of smell and taste we can experience. We are more likely to smell the popcorn of our fellow cinemagoers than anything we see on the big screen. Touch is occasionally added to digital devices, felt in the vibration of your cell phone; and while the fake shutter sound of your phone’s camera app gives you confidence that the shot has been taken, little else of the physicality of photography is incorporated.
It is instructive to note how contributions from the chemical senses go missing as we try to imagine every aspect of our lives being preserved online. This reveals just how neglected these senses are in everyday experience and how we have become hostages to our screens. That’s why each occasion when we step away from our screens to taste a wine and focus on the elusive nature of the precious liquid in the glass—its heady aromas, velvety texture, and persistent finish—provides an act of liberation, a blissful reminder of our embodied natures. These moments recruit us to what artist Olafur Eliasson calls the counter-numbness movement.
Could this outlook be too pessimistic? Is the absence of bodily senses from the digital landscape a mere technical limitation? Data fundamentalists and techno-optimists believe it is, firmly convinced as they are that vastly bigger datasets and more powerful algorithms will enable us to breeze through these limitations. The philosopher David Chalmers, in his recent book Reality +, is convinced. Virtual reality, he says, will eventually fill out these missing dimensions because “the physics engines that underpin VR” will continue to improve so that we will figure out how to handle the other senses. Chalmers believes that “like physical reality, VR […] can be a full-blooded environment for living a genuine life [… with] room for the full range of […] human [experiences].”1 He predicts that “within a century we will have [fully immersive] virtual realities that are indistinguishable from the non-virtual world.”
Chalmers and others are betting on advances in technology. But might there be reasons in principle to doubt there will be virtual wine tasting? I think there are. An experience of tasting requires us to put real liquids in real mouths, just as smelling requires putting real odorants up real noses. You might bolt on a delivery device to the VR headset that puffs wine aromas to the nose and squirts drops of liquid onto the tongue, but this still requires real odorants and liquids contacting real noses and tongues. Why go to the trouble of orchestrating real sensory experiences like these with what we see and hear via the headset when we could just give people a glass of wine? One reason we do not work and socialize more with each other’s avatars in the Metaverse is because these virtual meetings will contain no coffee and biscuits, no apéro at the end of the day.
Perhaps this is an unfair challenge. After all, we use our eyes to perceive the virtual bottles and glasses on the virtual table. Yet there is a crucial difference with smell and taste. For a virtual table to appear real, there must be a computer-generated image that causes you to see a table as you walk around it. And for there to be virtual aromas coming from the virtual glass, there would have to be a computer-generated entity causing you to smell it. But what sense could we make of a computer-generated aroma? Would a digitally created rose smell as sweet? For life’s most cherished experiences, what can’t be captured digitally will always remain essential.
Automating wine experts?
Even if the experience of wine tasting lies beyond the reach of AI, it is easy to see that talk about the wines we taste is digitally tractable. Wine writers and professionals put their knowledge and assessment of wines into words, and all this text can be mined for insights into what experts know and how that knowledge is organized.
Suppose machine learning techniques could be recruited to find patterns in the myriad tasting notes in the data training set to build a model that generated further tasting notes of the wines not mentioned in the training set. Could we train up an AI wine expert in this way to compete on equal terms with seasoned professionals? And would that system provide insights into the wine’s qualities or enable us to know what the wine tasted like?
A similar challenge arose in the world of perfume, and the attempt to address it provides a useful comparison with wine. As AI researcher Alex Wiltschko puts it: “Computers have been able to digitize vision and hearing, but not smell,” though he is convinced that “AI will revolutionize olfaction.” The aim of his digital startup, Osmo, “is to create digital representations of smell that will change how we capture, transmit, and remember scents.” So far, so good. The proof of concept came in a much-publicized recent paper in Science: “A Principal Odor Map Unifies Diverse Tasks in Olfactory Perception.”2 By using a deep-learning algorithm, Wiltschko and colleagues trained a graph neural network on a dataset of perfumers’ odor descriptions for single molecules to produce a model that maps molecular structure to olfactory perception—a key challenge in olfaction. Machine learning enabled the model to associate perfumers’ odor descriptions with vector representations for odor molecules starting from a training set curated from perfumers’ descriptions of more than 5,000 single molecules. Not only did the model recapitulate the odor labels the perfumers gave to these molecules, it could also extrapolate to new cases—specially created odor molecules that a trained human panel sniffed and labeled. In 53% of the novel cases, the model predicted odor descriptions for the molecules that more closely matched the mean performance of the trained panel.
The results are impressive, but there are important limitations when it comes to comparisons with wine. The deep-learning algorithm enables the model to generate odor-quality labels for odor molecules, but these are single molecules. Most odors we perceive are mixtures, and the perception of odor mixtures is notoriously hard to explain, both at the receptor level and at the cortical level of the olfactory bulb. Olfactory researchers Joel Mainland of the Monell Center and Richard Gerkin of Osmo are currently adapting the Principal Odor Map’s model to generate accurate odor descriptions for two or three odor combinations. At this point, however, it is worth remembering that wine aromas of any interest will contain more than 800 volatile odor compounds.
Importantly, the AI system that generates the Principal Odor Map is trained without ever sampling odors. No nose was involved in the building of this model. It produces a linguistic mapping from one representational format to another, learning to associate vector representations of molecular structures with linguistic descriptions produced by perfumers.
The trouble comes because researchers writing about digital olfaction have a tendency to elide the difference between odors and their labels. We are promised “a generalized map of structure-odor relationships,” though what we are really dealing with are computational proxies for these items. There is no sensing, which raises the question of whether an AI-generated structure-odor map can tell us how a molecule smells. It can if the model unlocks the relationship between a molecule’s structure and its odor and if the descriptions of its odor are a good guide to what we would smell.
What we find in the training set and the outputs are labels that practicing perfumers use to describe a molecule’s odor quality. Do such labels equip us to know how a molecule smells? If I tell you a given molecule smells creamy, floral, ethereal, and green, do you now know how it smells? I suspect not. Similar considerations apply to the tasting notes wine professionals use to convey how a wine tastes. As I argued in a previous issue of this title, a sip (or a sniff) is worth a thousand words.3
The fascinating question that got the whole inquiry off the ground was: What features of a molecule—or, for that matter, a wine—are experts picking up on when they classify their aromas or tastes? Can a model driven by a deep-learning algorithm illuminate what experts know? Perhaps—if the model latches onto the features of items in the training set that perfumers or wine tasters latch onto in order to perform the task. But is there any reason to suppose that AI systems use the same representations that human perceivers use? There is little reason to think so, but the truth is, we simply don’t know. How the system configures the layers between inputs and outputs, and what underlying structure deep learning imposes on the inputs to bring order to the odor landscape, remains opaque. An AI system generates predictions, but we still don’t understand how. It learns by picking up on statistical regularities between high-dimensional representations of molecules and human-derived odor labels. But beyond that, there isn’t much we can say. Like most deep-learning models, this system is a black box.
Wine chemistry and AI
This does not mean that we cannot learn about the key chemical compounds responsible for desirable aromas of, say, undergrowth, truffle, licorice, mint, and red and black berry fruits in the bouquet of aged red Bordeaux wines. The discovery that these aromas are more pronounced in certain combinations, and with certain concentrations of dimethyl sulfide, 2-Furanmethanethiol, and 3-Sulfanylhexanol, shows the possibility of discovering the chemical basis of typicity in these wines.4 This finding, however, was the result of painstaking profiling done by analytic chemists and sensory scientists, who compared Left Bank wines tasted and rated by a tasting panel with their chemical signatures. These fingerprints can be etched from finding patterns in the data produced by gas chromatography–mass spectrometry, where samples of wine are vaporized, separating them into their chemical compounds. The hope for wine AI would be to train a model to associate gas chromatography–mass spectrometry readouts for wines with a tasting panel’s aroma descriptions for those wines. The test of the model would be to see whether it could generalize beyond the chemical signatures of the wines it was trained on to generate aroma labels for other wines that coincide with the labels of the tasting panel. Is this possible? Time will tell.
More promising is the idea that AI systems trained on chromatograms can tell you which estate a wine comes from and what vintage it is. Proof of concept has already been established in a collaboration between Geneva neuroscientist Alexandre Pouget and Bordeaux wine chemist Stéphanie Marchand, who was involved in the study just mentioned.5 Pouget and his team analyzed the chemical composition of 80 Bordeaux wines from 12 different vintages. The wines came from seven different châteaux. The team then used machine learning to find patterns in the data for 73 of the wines, identifying them as coming from particular Bordeaux estates. Once the model was trained, it was then presented with chromatograms for the seven held-back wines, and it was able to assign, correctly, each of them to a particular château. The patterns it found also revealed a clear separation between wines from the Right and Left banks of the Gironde, and some evidence of vintage difference. In this way, AI provides proof of concept that the “combination of the soil, rootstock, variety, location, blend, and winemaking practices” really does have a chemical signal for individual wines or châteaux, irrespective of the vintage. Assemblies of chemical compounds, their similarities, and differences reflect the elusive concept of terroir, assuring us that there is something objectively there for proficient tasters to pick out. Does it mean that humans and machines are picking out the characteristic similarities and differences between these wines in the same way? That’s unlikely. Again, we still lack any real understanding of how models built on deep learning work. What we do know is that there is something distinctive about these wines that the machine and the human perceiver can both pick up on in their own ways. In the future, we may be able to replace blind tasting with machine learning.
Wine apps versus wine experts
Coming back to the present day, the widespread use of wine apps is perhaps the most obvious application of digital technology to the world of wine. How transformative has it been or might it become? At the start, wine apps like Vivino and CellarTracker provided a form of cognitive off-loading, an outsourcing of our judgments of which wines to buy. The phenomenon isn’t new. Consumers faced with a bewildering range of wines on offer in a store often turned for advice to authoritative wine critics who gave points or scores. Increasingly, they have turned to wine apps to guide their purchases. Digital technology was there to replace reliance on expert wine tasters whose judgments did not line up with ordinary wine consumers’ tastes and preferences.
Wine apps are recommendation systems, like those used by Amazon and Netflix, that tell you that people who liked X also like Y. Their recommendations are based on a “wisdom of the crowd algorithm,” guided by what most people like, or what people like you like, without delving into why you like it. The “people like you” part is important because it takes us beyond the old joke of a machine-learning algorithm going into a bar where the barman says, “What are you having?” and the machine-learning algorithm replies, “What is everyone else having?” Recommender algorithms rely on the idea that there is enough data in consumers’ purchasing patterns and preferences to extract reliable predictions about what else they will like. The first task is to crowdsource data from consumers’ ratings, then generate predictions through statistical averaging over the inputs. Apps such as Vivino offer aggregate scores for wines whose labels consumers have scanned, along with personal recommendations by users; CellarTracker pools the scores of thousands of community reviewers and lists points, prices, and availability of wines.
The declared advantage of these systems is twofold: (i) algorithms that aggregate the opinions of others will serve better than relying of the opinions of a few renowned wine critics; and (ii) subjective liking is a sufficient basis for wisdom of the crowd judgments.
On (i), the opinions of wine critics are said to differ from those of ordinary consumers because they perceive the taste of wines differently, or diverge from everyday wine consumers in their preferences, so they don’t like the same wines. It is a regular trope that “customers lacking a wine education actually prefer cheaper wine,” and we hear that wine merchants “have to listen more attentively to those consumers”—who usually buy at the low end of the market—“rather than continuing with the current approach of telling them how much better more expensive wines are, even if they do not happen to like them so much.”6
When it comes to (ii), the wisdom of the crowd is meant to explain why the collective opinion of a group, as the aggregated answer to a question of quantity, is superior to the answers given by individuals in the group. When asked to estimate the height of the Eiffel Tower, or the weight of a bull at the fair, in Francis Galton’s example, people will make wildly different estimates, but when the average of the estimates is calculated, the result is often nearer the right answer than the individual estimates. The variation between the answers is smoothed out by averaging, which eliminates noise. If we accept, however, the subjectivity of taste—that no opinion is better than any other, and that all opinions are equally valid (or invalid)—and if individuals are just expressing their own liking, it is hard to see how the aggregated liking score corrects for errors or eliminates noise. To what does the collective decision more accurately correspond? Why should I value the opinion of the crowd over my own if all opinions are equally valid? Because, it will be said, the aggregated scores will predict what I will like based not on the wisdom of the crowd, but the preference of the crowd. Often these are frequently purchased wines with high average liking, where whether I like a wine will be based on how much that wine is liked by people who overlap with me in their judgments of wines I have previously tasted (and rated). The more similar I am to others, the more they can serve as guides to what I will like. These are the arguments for the widespread adoption of recommender wine apps.
But will averaging of consumer liking at a given price provide the individual with anything useful on which to build or extend their wine knowledge? Is there a way to go beyond mere averaging? Is there, more importantly, a way not only to help people look for information about a wine they know, but discover new and interesting wines?
There are basically two approaches to building wine recommendation systems: collaborative filtering and content filtering. The former harvests data from consumers, finding patterns among their “likes,” purchases, and any recommendations for others, giving greater weight to similarities and predicting liking on the basis of overlaps in taste. This can also be done with wine items rather than app users. Recent matrix factorization techniques use machine learning to arrive at abstract features characterizing both users and wines, although the features used by the system to generate taste predictions remain utterly opaque.
Content filtering, by contrast, is based on features of the items liked, reviewed, or purchased, such as grape variety, country, region, whether biodynamic or not, and it involves seeking other wines to recommend that share these features. Once enough ratings have been harvested, it is possible to assess the importance of such features for target users. Thus, an invaluable source for content-based filtering systems comes from the tasting notes that consumers offer to describe wines. Tasting notes can provide the linguistic raw data to which recent natural language processing techniques can be applied.
Predictions of consumers’ tastes are even more accurate when combining these filtering methods in hybrid recommender systems. For example, we can have a collaborative-filtering algorithm and a content-based algorithm generate independent predictions and then aggregate their predictions through the use of a hybrid algorithm.
Extending the search space
The power of these algorithms when applied to big datasets is impressive, but how well will these wine apps serve consumers who are looking to extend their knowledge and experience of wine? How far do they depart from the assessments of wine experts? And how could they be improved?
The first question has mixed answers. As music artist David Byrne once put it, describing music recommendation apps, they tend to produce memes, not masterpieces, and the same may be true of the wines to which the most popular apps direct drinkers.
At a recent conference on judgment at Oxford’s Institute for Ethics in AI, I conducted a tasting experiment on more than 100 people with no special expertise in wine tasting. They were given a glass of French Sauvignon Blanc to taste and then offered two other wines to sample, both Sauvignon Blancs. They were asked to decide which wine they would suggest to someone who liked the first wine. Nearly all of them chose the second wine, also a French Sauvignon Blanc, while the Vivino app recommended a slightly more expensive New Zealand Sauvignon Blanc. What these drinkers were able to judge better than the app is how alike wines are, while what the app is designed to do is nudge people to wines in the same broad category but in a slightly higher price bracket. It is no surprise that wine apps are often developed with wine stockists to help take people on their “wine journey.” But while a wine writer may suggest white wines from the Rhône to white wine drinkers who don’t like too much acidity, and recommend a Condrieu to drinkers in this group who want to try an exceptional wine of that type, it is surely unlikely that the app will go out on a limb.
As for the distance between the ratings of wine apps and wine experts, things are not as straightforward as they first seem.7 In a comparison carried out for Vox magazine in 2018, Mark Schatzker and Richard Bazinet found for 9,919 California wines a Spearman correlation of 0.576 between the ratings on the crowdsourced app CellarTracker and those in Robert Parker’s Wine Advocate; and for 1,099 California wines, a Spearman correlation of 0.424 between the ratings of CellarTracker and those on Jancis Robinson’s website. What is the explanation? CellarTracker’s founder Eric Levine notes that the average user has rated 49 wines and that 2,311 users have rated more than 500 wines. So, these are not novices making speculative estimates about a continuous quantity like the weight of a bull or the height of the Eiffel Tower. It is unlikely that they are merely offering personal likings to be averaged either, since there is more convergence of opinion for higher-priced wines. Like expert tasters and wine professionals, the individuals in this crowd are not judging their personal liking, but wine quality.
Wine apps search for patterns in the likings and purchases of consumers, predicting which other wines they will like, but they cannot tell us anything about the basis on which consumers like those wines. As we’ve seen, a machine-learning algorithm is a black box that offers no explanation for the generalizations it makes.
The absence of insight into liking leaves a gap, however, that can be filled by wine experts, such as winemakers, wine merchants, sommeliers, and writers. Wine experts may not share the personal tastes of everyday consumers, but they do have insights into what unifies the range of bottles a consumer has purchased, rated, and recommended to others. Experts are more consistent in their judgments than novices, they discriminate more finely, and this can be harnessed to discern the features in the wines everyday consumers like.
Expert-augmented datasets
In work carried out by my colleague Ophelia Deroy and computer scientist Pantelis Analytis, they asked whether the advantages experts have in terms of consistency and density could outperform the wisdom of a larger, more diverse crowd if their ratings were fed into a collaborative-filtering recommendation system.8 Bordeaux wines are a good test case. They are rated by experts and enthusiasts from all over the world. Of course, experts engage in regular and extensive tastings, but their ratings also provide denser datasets to be explored by data scientists. Looking at our selection of Bordeaux wines, amateur data remains sparse in comparison, with about 120 individual users kept in the sample since they had evaluated at least 50 wines each in their profile. The data harvested from the en primeur tastings of 13 renowned experts was much denser at the individual level, which is not surprising, since amateurs do not often attend the en primeur tastings of newly produced wines.
Using a collaborative-filtering algorithm, they first compared the taste of wine experts to those of enthusiasts active on social wine platforms for some 2,000 Bordeaux wines. True, the results confirmed a gap between experts and app users; unless you are an expert, your taste in Bordeaux is likely to be more similar to that of other enthusiasts than to that of Jancis Robinson MW, Robert Parker, or Decanter wine critics. Experts, however, showed more consistency than enthusiasts; their ratings of Bordeaux wines are comparatively more congruent with each other than the enthusiasts’ are with each other.
So, what happens when we combine both sets of ratings? For our comparative test, a recommender system where people (both experts and consumers) could draw advice only from experts performed better in terms of prediction than a recommender system where people could draw advice only from consumers. In both systems, we identified the most similar experts and the most similar consumers, and the opinions of the most similar others were then consulted for the test items. Using collaborative filtering, most people are better off receiving recommendations based on data collected from a dozen critics than from a group of more than 100 consumers. The difference may be modest, but in prediction problems, even a small edge can create a large market impact.
Wine apps such as Vivino, CellarTracker, and their competitors will try to improve their systems by getting more everyday consumers to provide more ratings and reviews, and to apply collaborative-filtering and content-based approaches, or their hybrids, to improve recommendations. Systems like Preferabli rely on huge datasets and content-filtering algorithms drawing on mainly Master of Wine- and Master Sommelier-based expert assessment to find wines that meet the personal profile each user builds up. A genuine collaborative filtering, however, could be one that filters out neither the taste of experts nor enthusiasts but combines them to good effect.
Augmenting the consumer data with expert data could predict enthusiasts’ tastes better than just the enthusiasts, or the experts, alone. Rather than collecting more amateur ratings and deploying more sophisticated recommender algorithms, another way forward would be first to bring together ordinary consumers and wine experts on the same platforms. Even though your taste is most likely different from that of experts, you still benefit considerably from bringing them in because of their consistency, and their broader wine coverage will give them a better ability to predict novel wines that you will like. The specific benefits of aiming at an expert-augmented dataset should not be missed. Combining the ratings of experts and amateurs could help to bridge the distance between professional judgments, aiming at shared judgments of quality, and the personalized recommendations that consumers seek.
Going beyond merely improving predictions, getting recommendations from a blend of expert and amateur ratings may, in the future, produce better recommendations and eventually also unlock new opportunities to exercise and refine people’s tastes by picking out truly novel and more diverse wines. Maybe that is the future. Let’s hope so.
Notes
1. David J Chalmers, Reality+: Virtual Worlds and the Problems of Philosophy (Penguin, London; 2022), p.xvii.
2. Brian K Lee et al, “A Principal Odor Map Unifies Diverse Tasks in Olfactory Perception,” Science 381 (2023), pp.999–1006.
3. Barry C Smith, “Is a Sip Worth a Thousand Words?” WFW 21 (2008), pp.114–19.
4. Magali Picard, Cécile Thibon, Pascaline Redon, Philippe Darriet, Gilles de Revel, and Stéphanie Marchand, “Involvement of Dimethyl Sulfide and Several Polyfunctional Thiols in the Aromatic Expression of the Aging Bouquet of Red Bordeaux Wines,” Journal of Agricultural and Food Chemistry 63 (2015), pp.8879–89.
5. Michael Schartner, Jeff M Beck, Justine Laboyrie, Laurent Riquier, Stéphanie Marchand, and Alexandre Pouget, “Predicting Bordeaux Red Wine Origins and Vintages from Raw Gas Chromatograms,” Communications Chemistry 6 (2023), Article number 247.
6. Steve Slatcher, “Subjectivity in Wine Appreciation,” WFW 51 (2014), pp.136–41.
7. Barry C Smith, “Getting More Out of Wine: Wine Experts, Wine Apps, and Sensory Science,” Current Opinion in Food Science 27 (2019), pp.123–29.
8. Pantelis P Analytis, Karthikeya Kaushik, Stefan Herzog, Bahador Bahrami, and Ophelia Deroy, “A Recommender-Network Perspective on the Informational Value of Critics and Crowds,” https://arxiv.org/html/2403.18868v1#bib (2024).